A Survey of ML Techniques in Adversarial Image Forensics
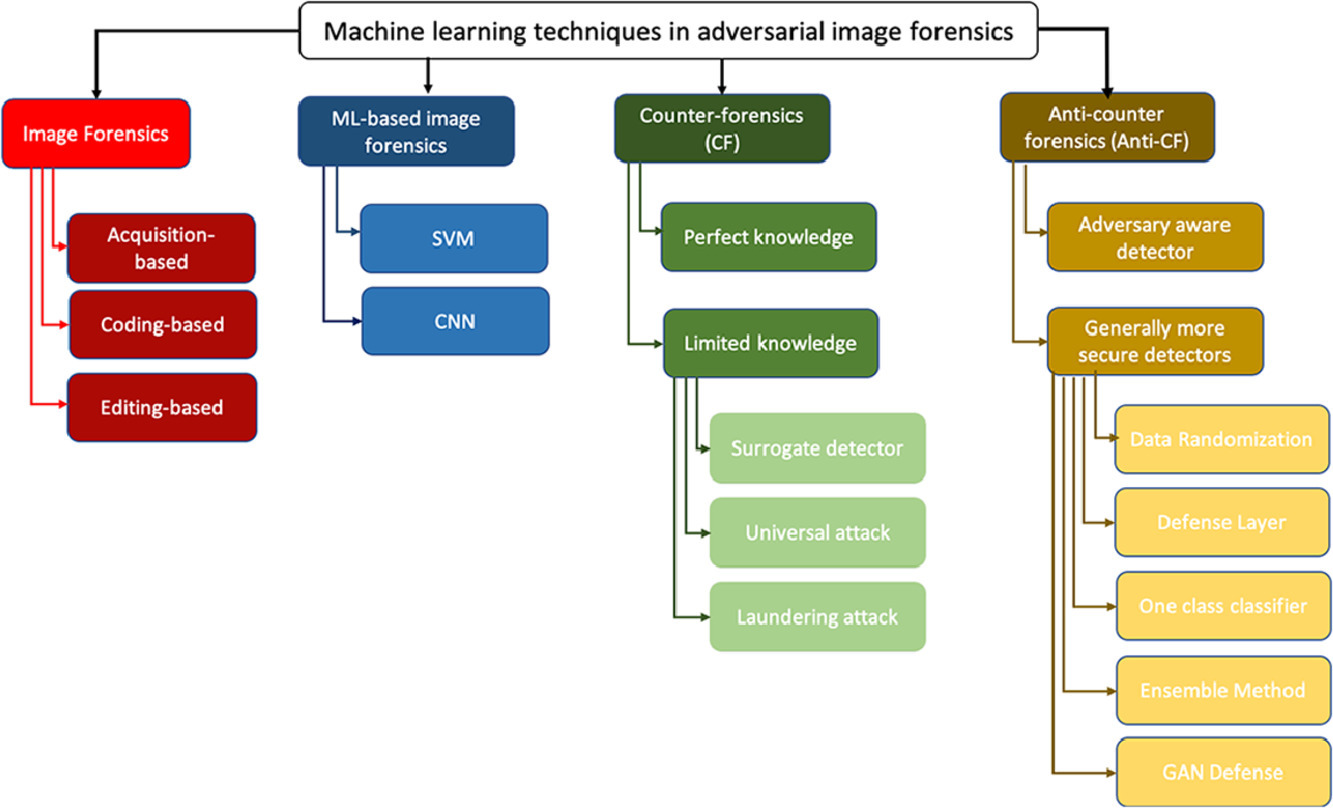
A number of literature surveys and reviews on the applications of ML-techniques in image forensics has been published in the literature and in image forensics, although adversarial image forensics is generally not discussed. Amodei et al., for example, reviewed the general security concerns in artificial intelligence, particularly reinforcement learning and supervised learning algorithms. A general review of security implications on the use of ML approaches and their countermeasures was presented in [8, 10]. Akhtar et al. focused on adversarial attacks on deep learning approaches in computer vision. However, there have been limited studies focusing on ML-security issues in (adversarial) image forensics, a gap we seek to address in this paper. Specifically, in this paper we survey existing ML techniques for image forensics, including those that can be utilized in the adversarial setting (e.g., image manipulation), and CF. In the survey, we also reviewed the various approaches that can be used to enhance the security of binary manipulation detectors based on ML and defensive techniques during the testing stage. Figure 1 shows a graphical abstract concerning the application of machine learning techniques in adversarial image forensics.